9000 Plans
[ Housing design through machine learning American residential plans ]
The project began with an exploration of the deep residential plan and generic housing projects in America, where the sheer quantity of developer-driven condominiums created a normative lifestyle tradition.
We deployed a machine learning algorithm called StyleGAN to find the internal visual logic behind these unit plans.
This AI reads the dataset as pixels, and produces new floor plans from noise images and pre-trained data of human faces. The learning process transformed machine-generated faces into machine-generated floor plans.
2021 Spring
GSD Core 4
Instructor: Jennifer Bonner
Teammate: Justin Ng, Zixuan Luo, Ruiheng Li
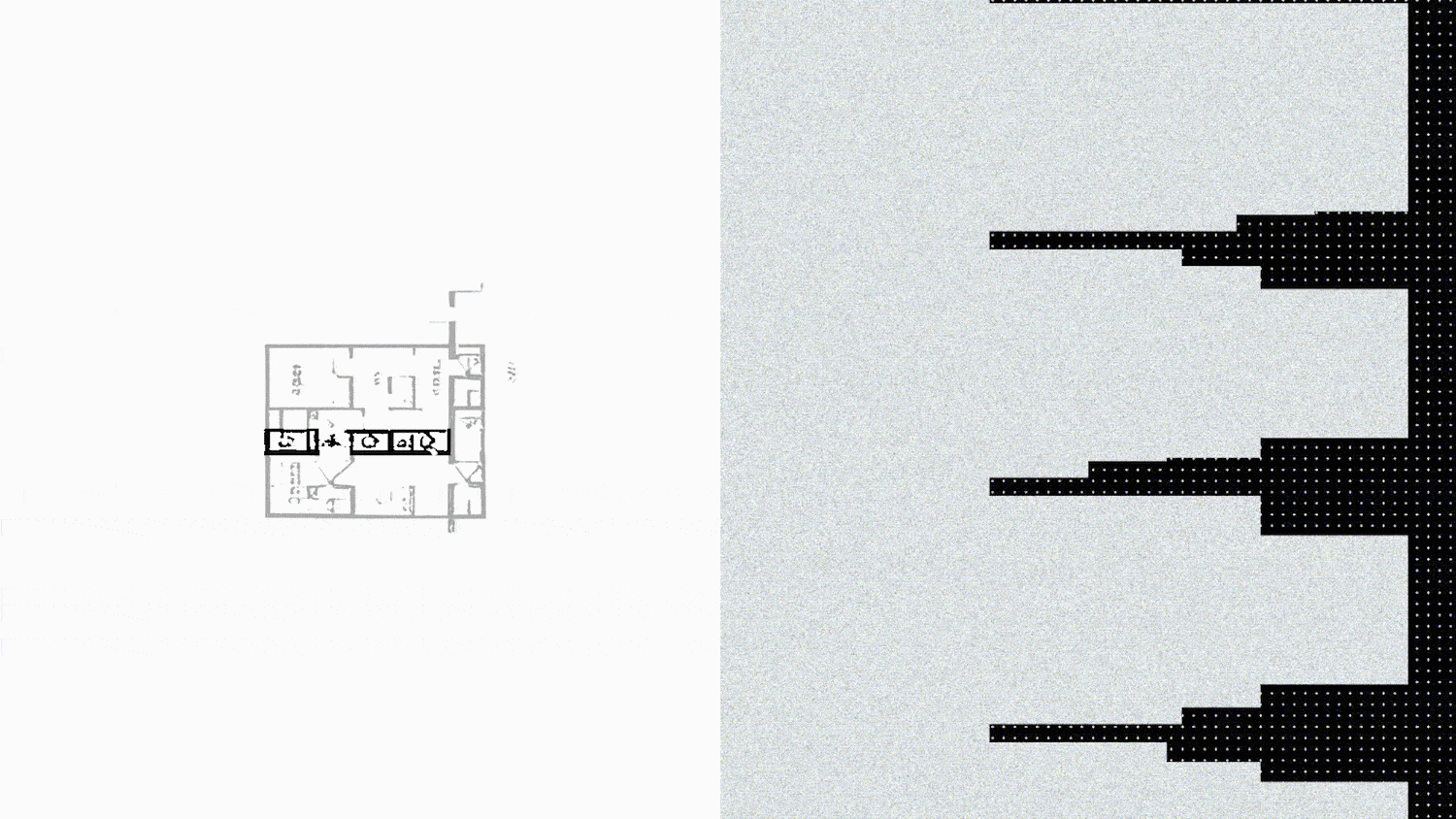
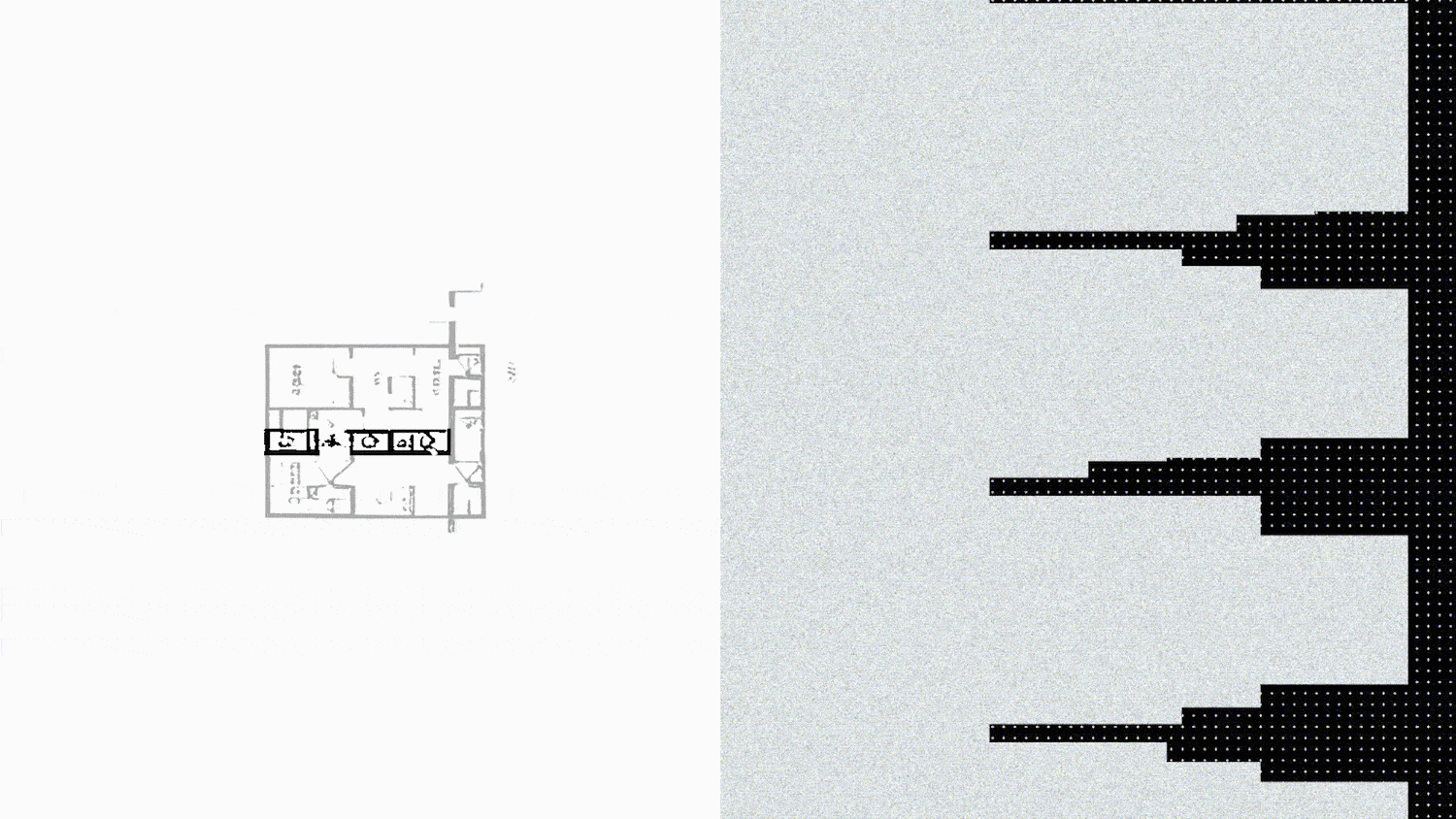
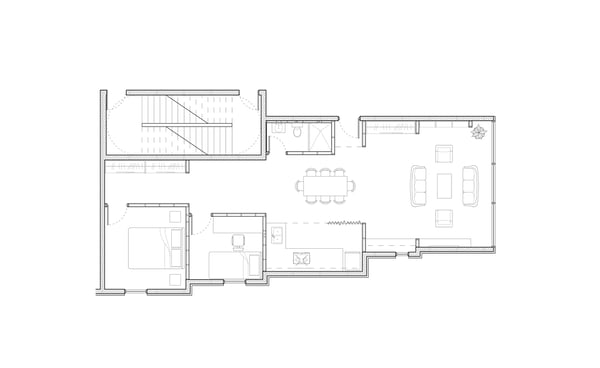
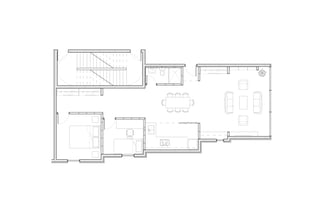
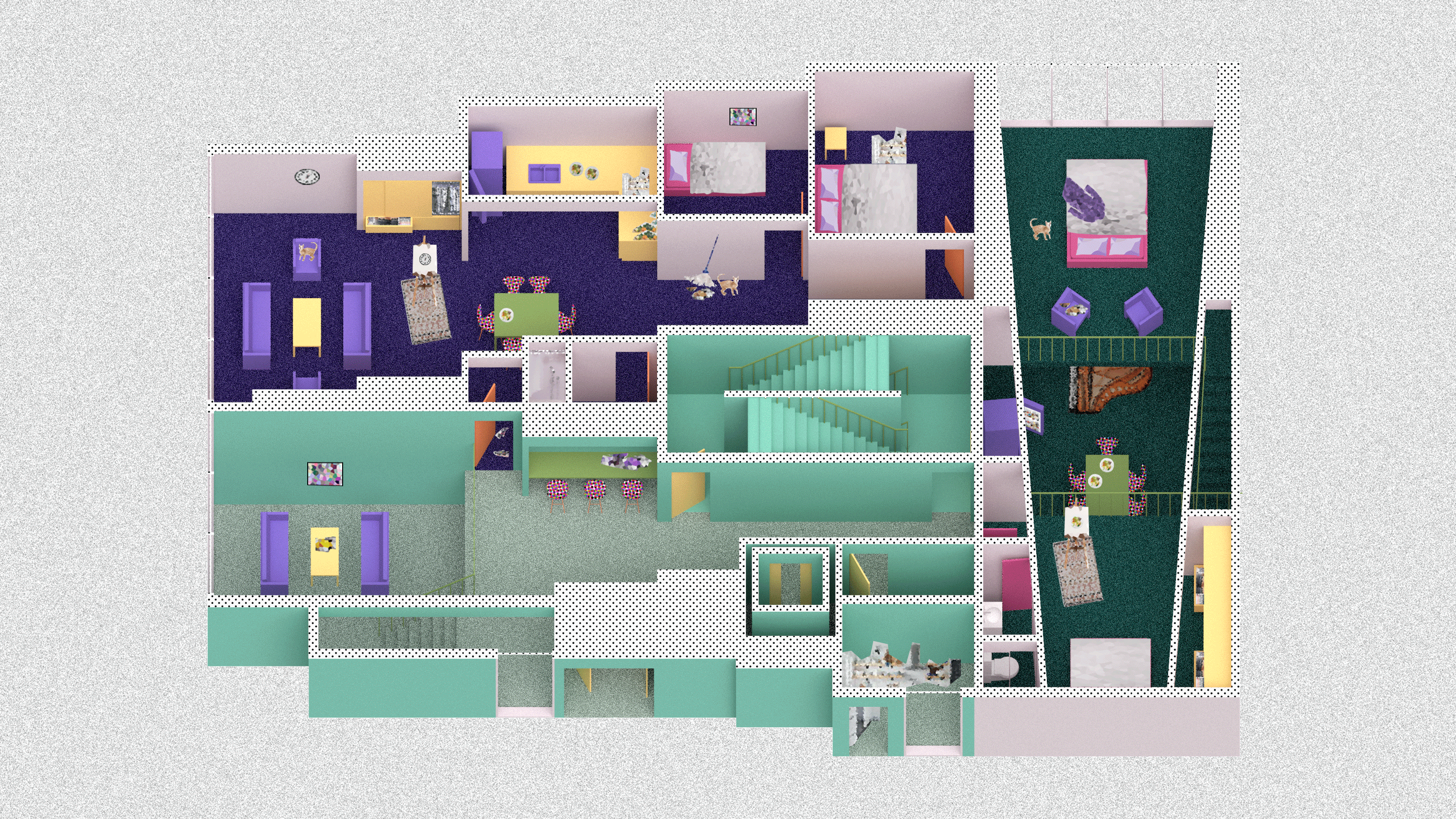
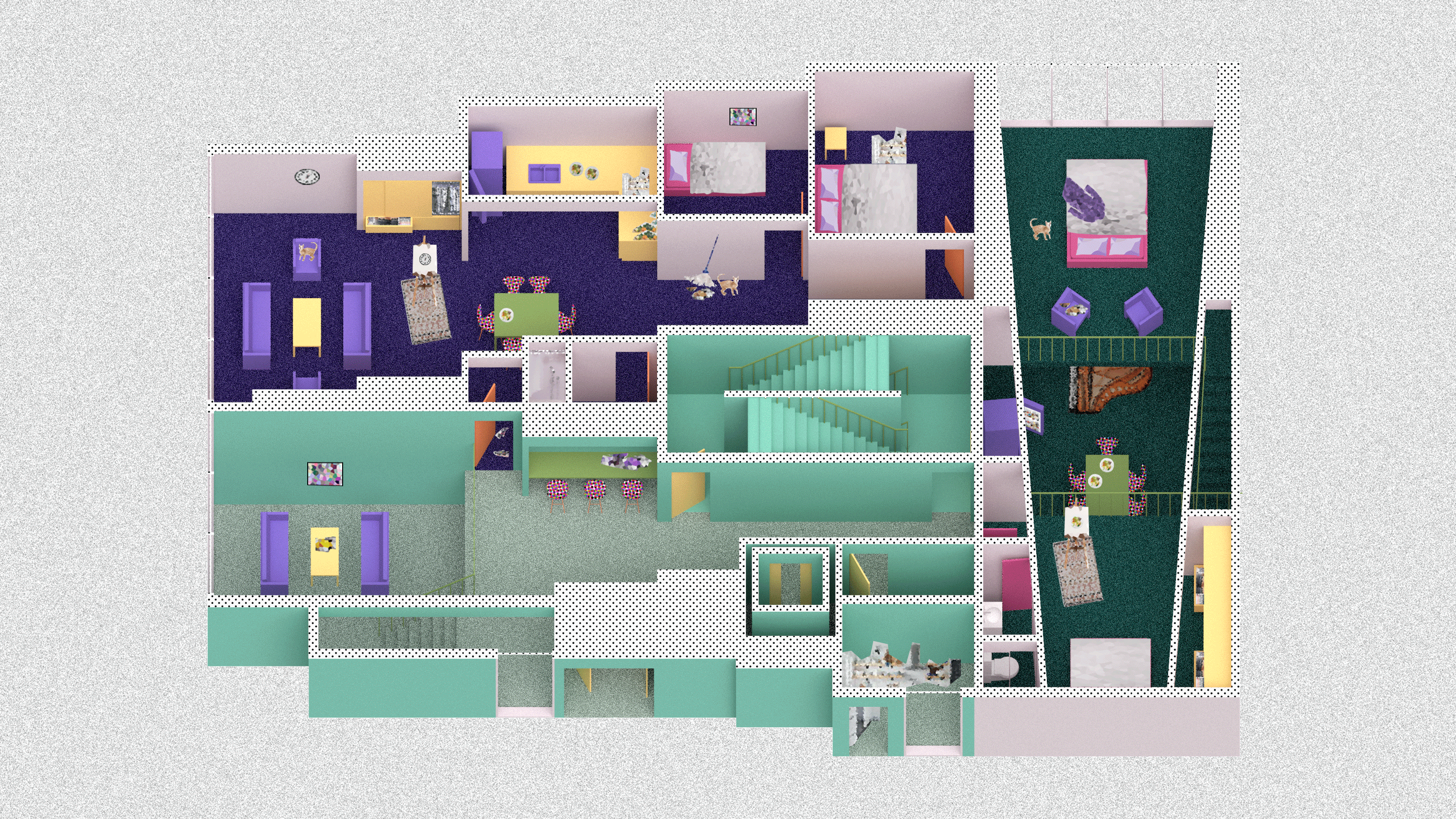
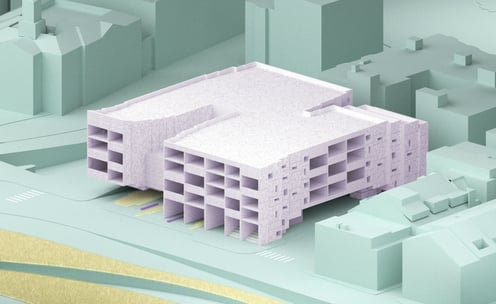
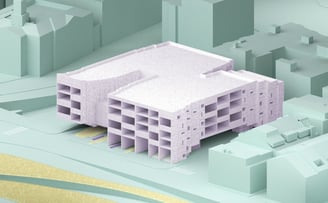
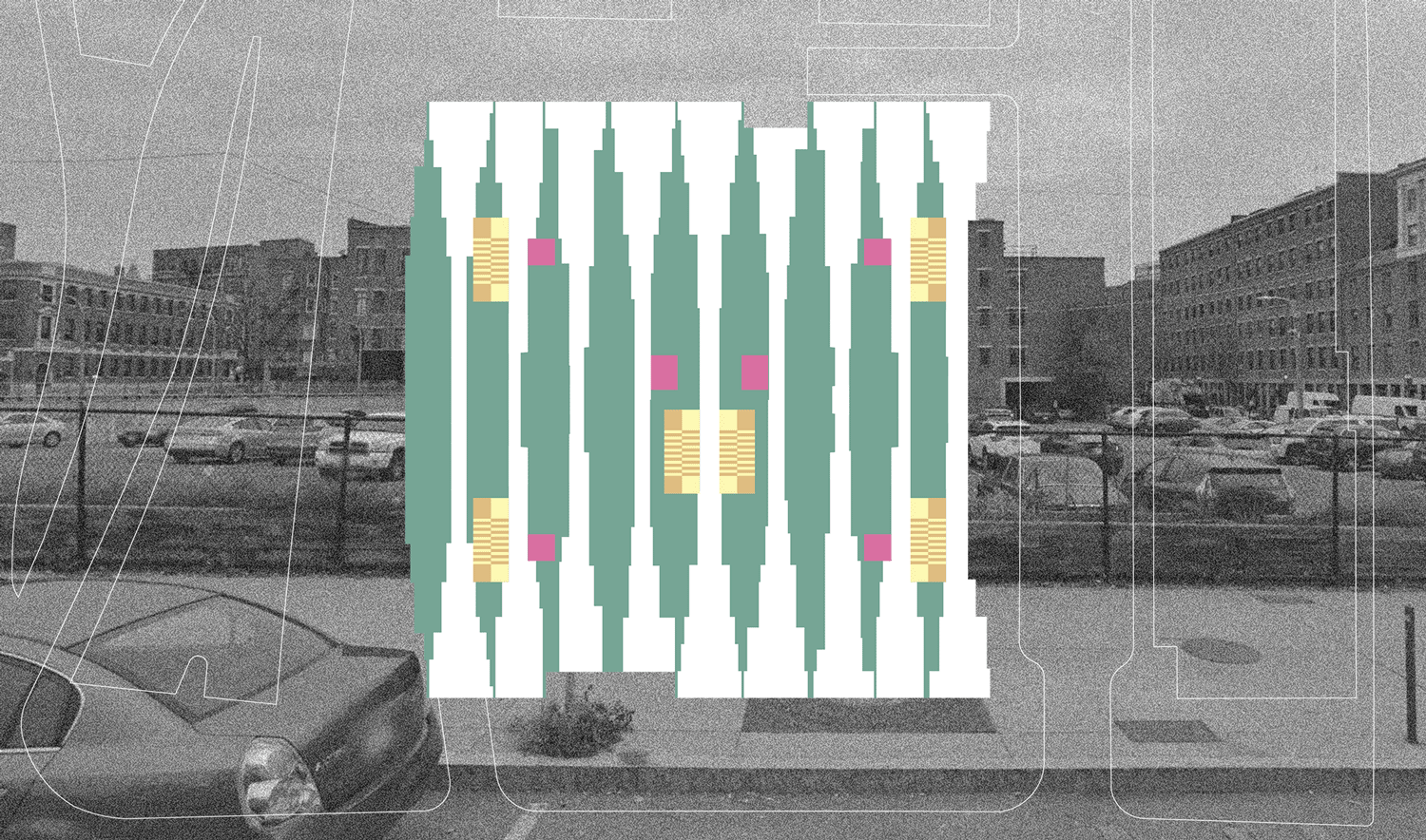
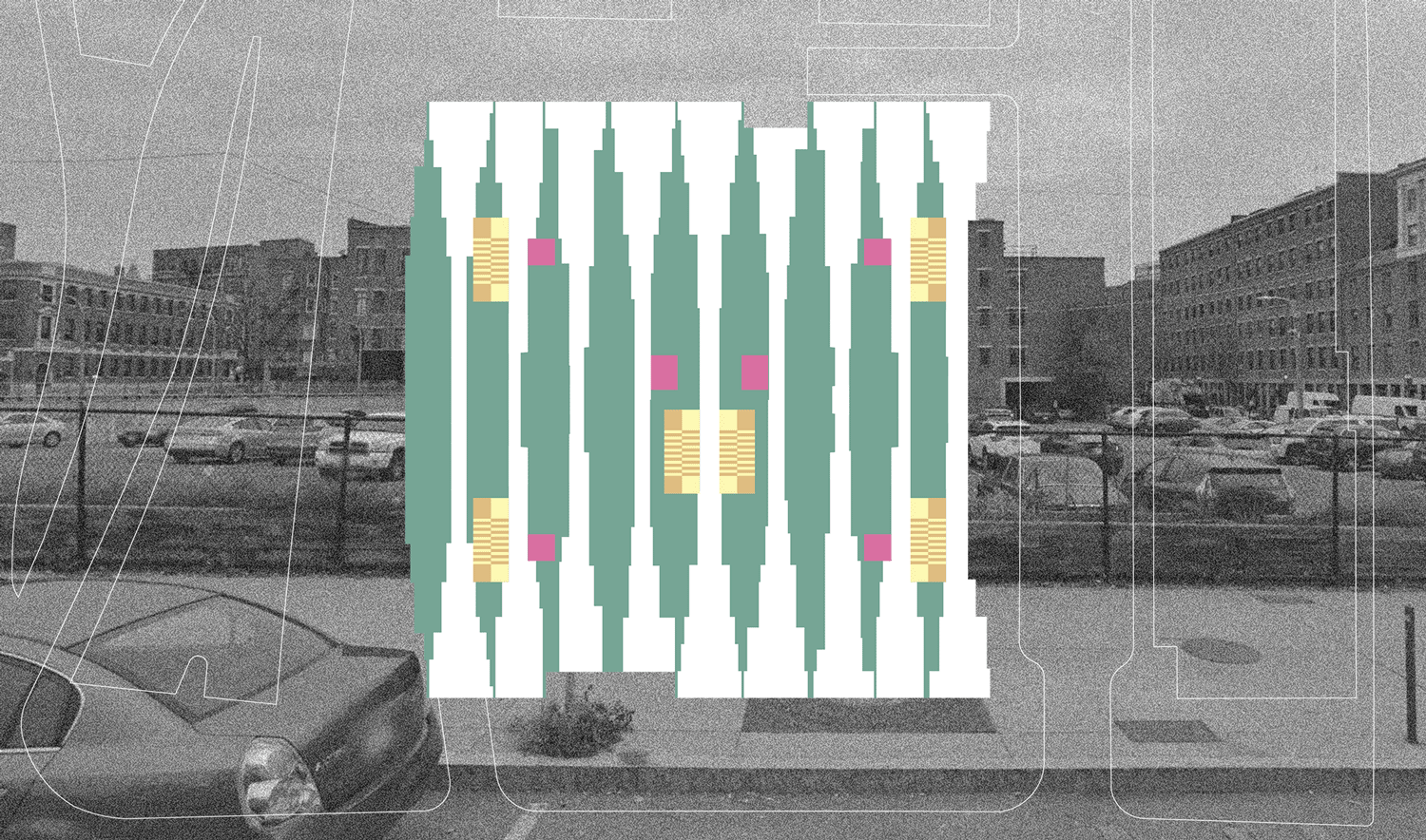
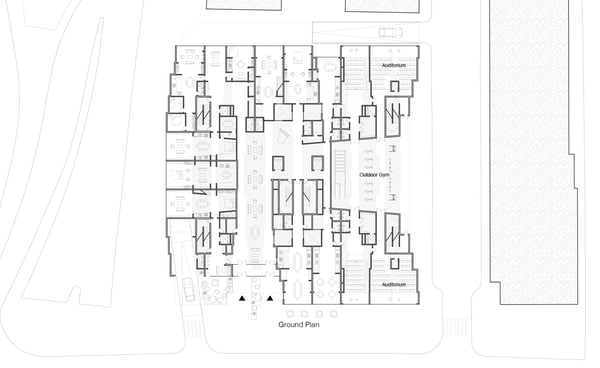
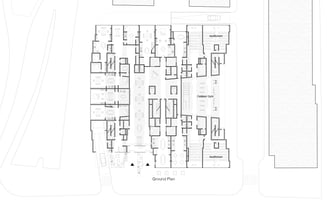
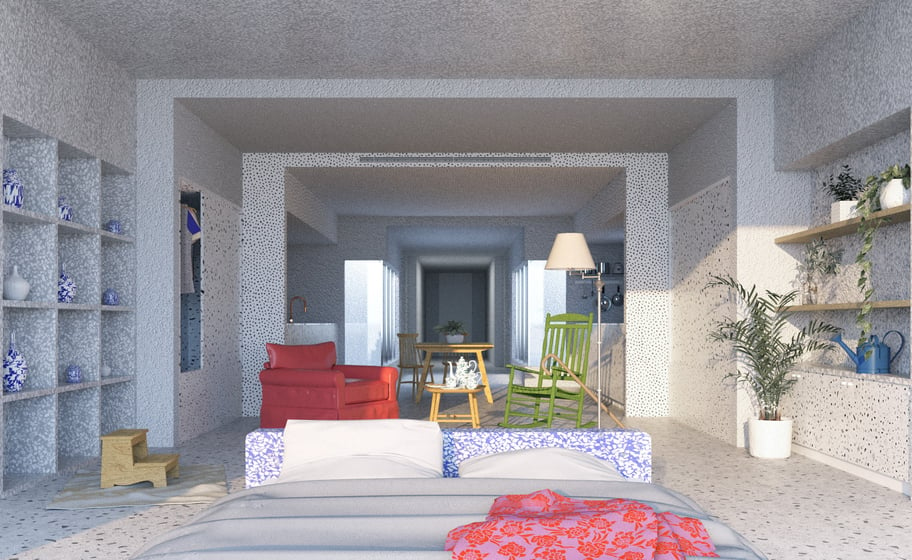
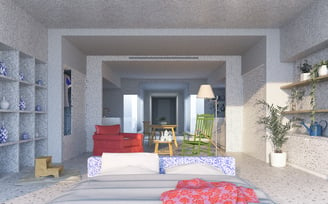
Units on the square site emerged through discretizing a gradually widening bar and the resulting stepped figures becomes a place for stuff like wardrobes, books and kitchens…
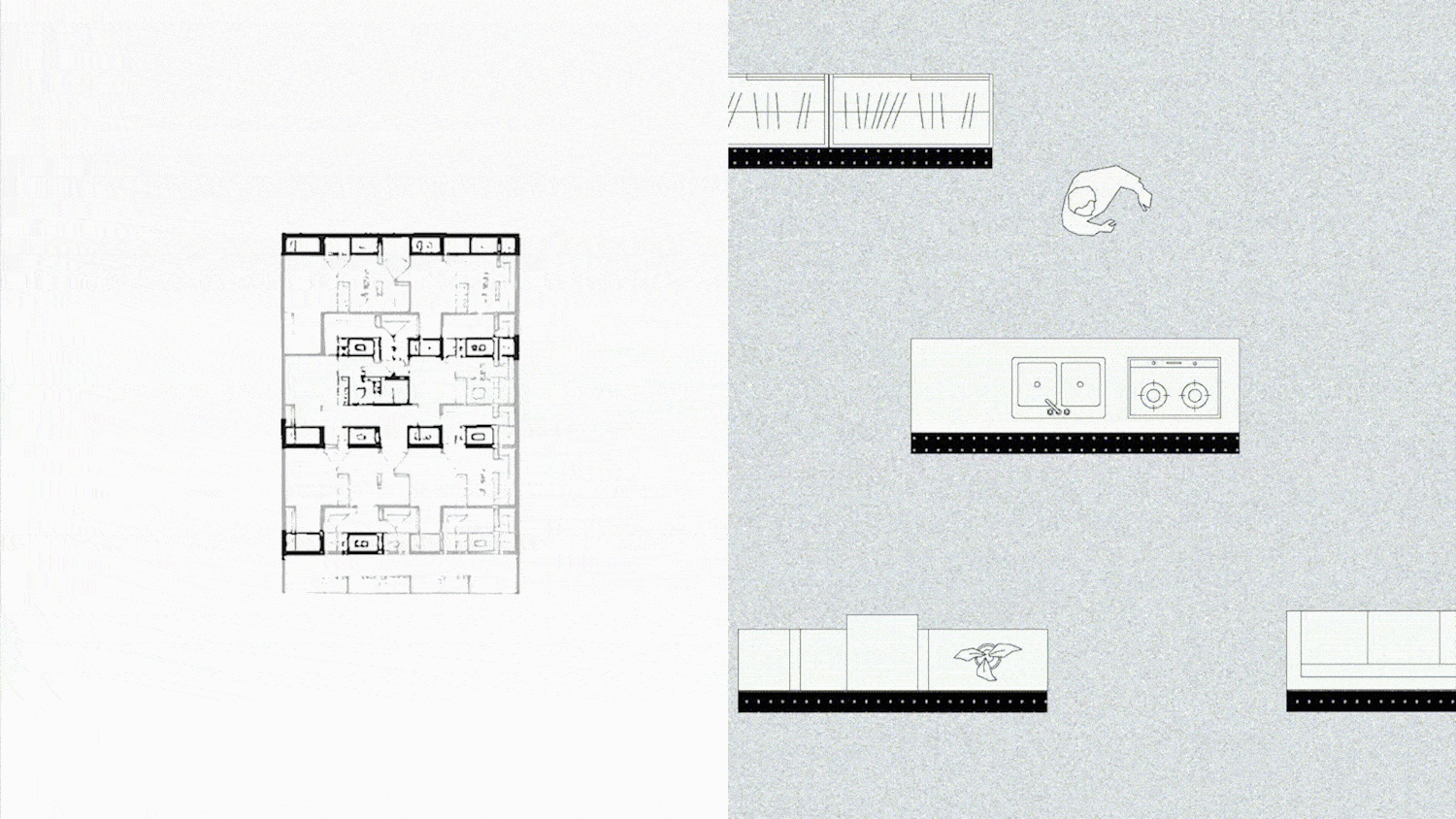
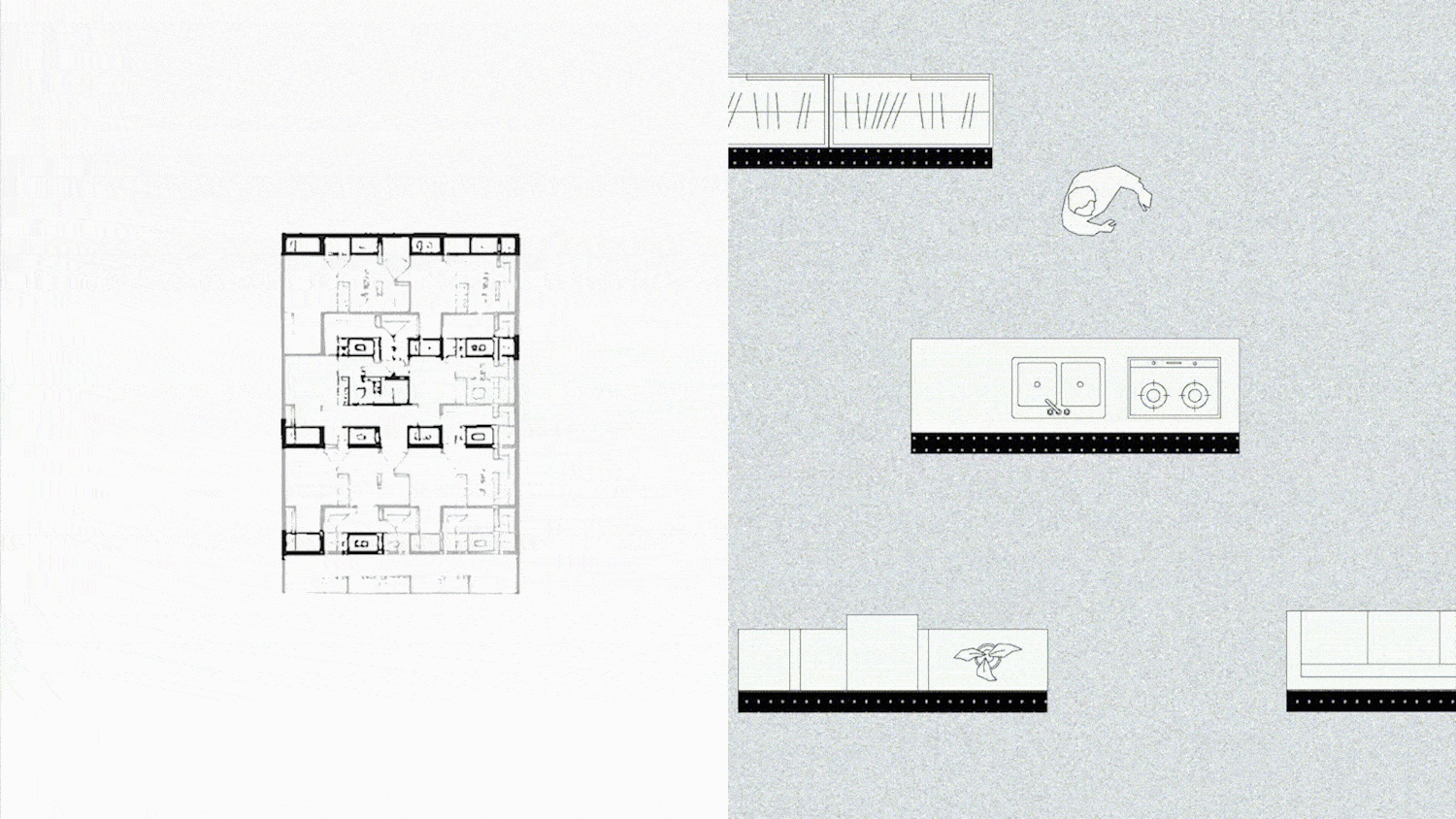
Site A
Site B
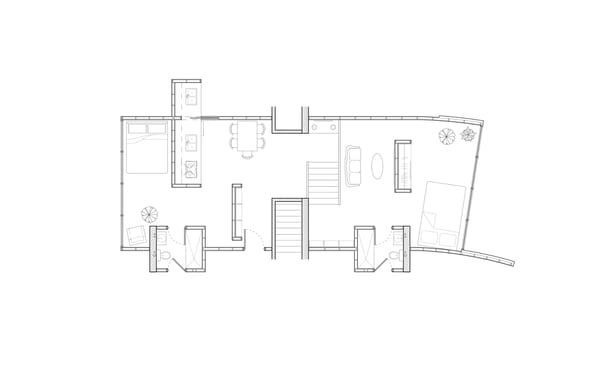
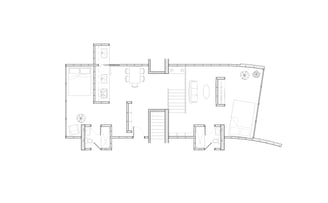
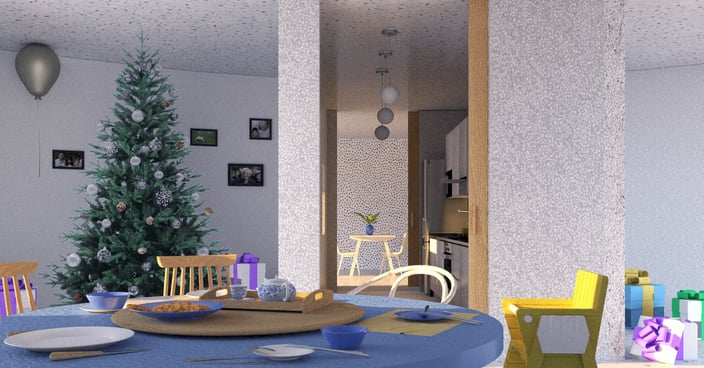
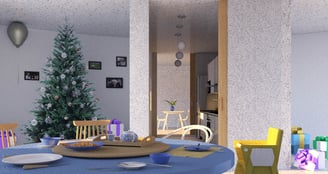
The triangular site’s design emerged from another interpolation of the machine’s recurring bar figure, where bars are also filled with stuff.
Yet unlike the other building, these bars run perpendicular to the units and act as filters that choreograph spatial hierarchy.
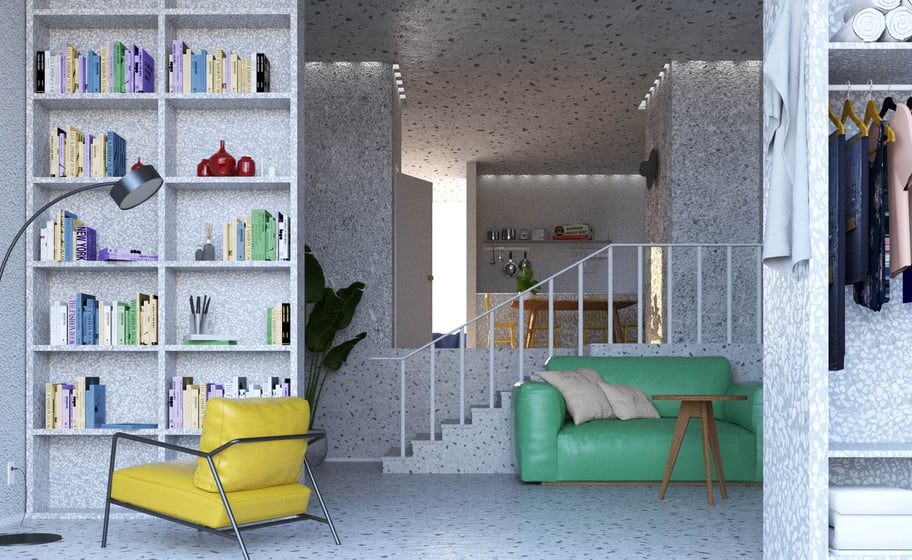
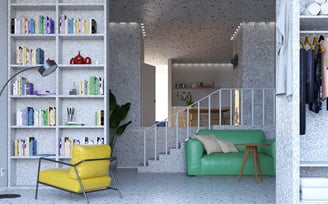
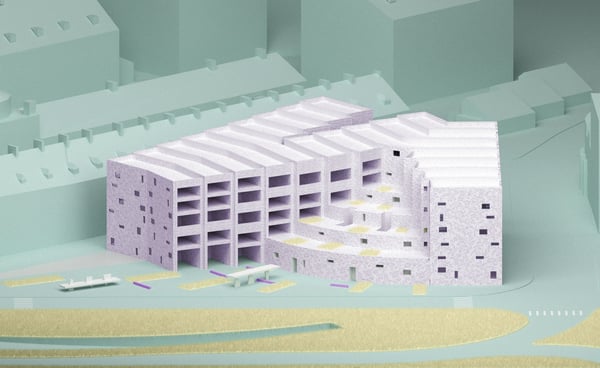
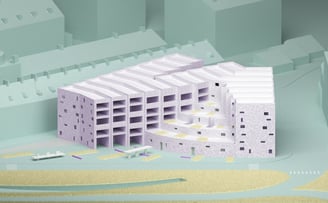
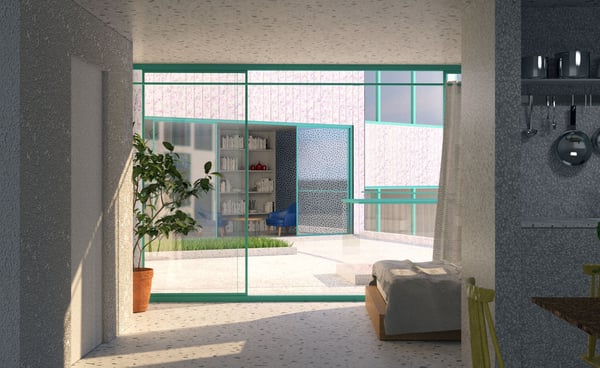
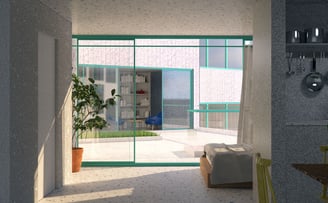
Intersecting unit boundaries, these blocks are shared and produce programmatic enfilades. The enfilade’s door alignment introduce a curiously new collectivity that can expand and contract to different families’ desire.
The pivot visually delineate two ends of a unit without imposing a hard boundary. They foster a second type of multigenerational units, where two generations share a outdoor terrace.
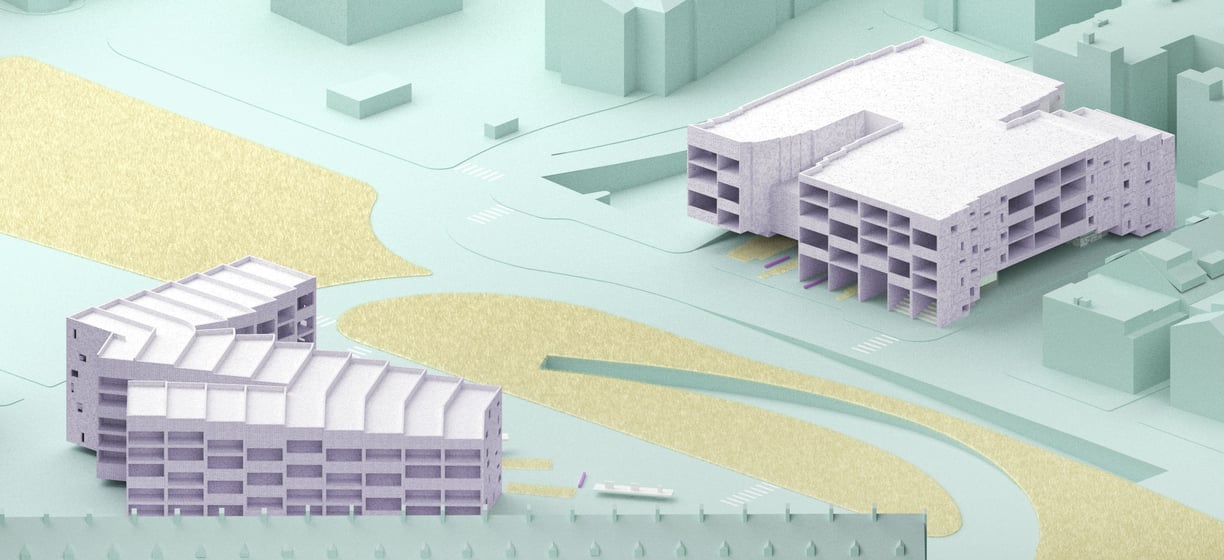
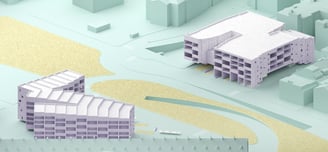
Two buildings that emerged from independent interpolations of the machine-learning results match across the greenway like two bent bars facing one another.